In this age, almost every other individual uses AI text generators to make blog posts, write letters, send emails, write research papers, and so on. However, there are some sectors that ask for original and human-written text, such as respected academic institutions and some legal institutions. Additionally, giant search engines value written content written by humans. Therefore, it is important to write content manually. However, the challenge arises when the human-written content is marked as AI-generated by famous AI detectors.
To this, we say false positive AI detection. It is disappointing to learn that your text is highlighted as AI-generated when you have written each and every word yourself. Why does this happen, and how can this false positive AI detection be avoided? If you have ever come across such a situation, this is troublesome. Don’t worry. In this guide, we will discuss all the possible methods to write a great piece of text and escape AI false positive detection.
What is a False Positive?
AI detection systems are more common now. Many of these systems have problems with false positives. This problem happens in many areas. These areas include text, images, videos, and behavior analysis.
- Textual content: It is hard for AI to find misleading or harmful information in text. Sometimes, safe content can be wrongly marked as harmful. This happens because the system does not fully understand context or sarcasm. When this happens, users feel frustrated. They also lose trust in AI technology.
- Images and videos: Detecting inappropriate material can also create false positives. AI algorithms can mistakenly flag harmless images as inappropriate. This happens because the AI does not understand the context of visual elements. As a result, harmless visuals may get labeled as harmful. This causes problems for users.
- Behavioral analysis: AI often has trouble telling normal behavior from suspicious behavior. Sometimes, these systems label regular activities as dangerous. This creates false alarms. When this happens, it can confuse people who are just doing normal online activities. It also reduces the effectiveness of AI monitoring.
Causes of False Positives in AI Detection
There are three main causes of false positives, and these raise the question of whether AI detectors can be wrong.
Algorithmic limitation: False positives often come from how algorithms work. AI systems learn from training data. If this data is not complete, AI does not see the full picture. It only sees part of the data and makes mistakes. Bias in data can make the problem worse. If the data has only one type of example, the AI makes a bad model. This causes the AI to mistakenly identify people or actions.
Human errors: This also has a big role in false positives. Operators can wrongly identify content when they analyze it by hand. When humans do not see the bigger picture, even the best AI detectors can also make mistakes. Bad labeling practices confuse the systems, too. If a person labels data wrong, the AI learns the wrong thing. This means the system keeps making false positives.
External factors: The external factors also add to false positives. Noise in the data, such as random mistakes or extra information, can change what the AI sees. These distractions create wrong conclusions. Changes in the environment are important, too. For example, a change in social norms can make an AI misjudge acceptable behavior.
These factors work together to make a hard situation. As technology grows, it is very important to deal with these problems. To make sure AI is accurate, we need to think about data quality and human help.
How to Identify False Positive
Finding false positives is very important in AI systems. There are several ways to find these mistakes.
- Anomaly detection techniques: These techniques find patterns that are different from normal ones and which cause hallucinations in AI. By spotting these differences, systems can better identify possible mistakes. They can find times when AI wrongly marks normal data as suspicious.
- Cross-validation: This method uses several AI systems to check the same data. If one model marks an item while others do not, there is a chance of a false positive. By combining information from different systems, users can reduce incorrect alerts and make better AI systems.
- Case Studies: Case studies in real-world industries show how identification works. For example, healthcare technology handles patient data. AI detects anomalies that may signal the wrong medication. In this case, the AI system identified a possible error that a human missed. This event saves a life and proves the model’s reliability in finding errors.
In finance, another example helps us understand false positives. A fraud detection algorithm marks many normal transactions as suspicious. This happen because the model learned from biased data. After realizing the error, the company improved its system. It uses better data and adjusts its approach. These changes lead to fewer false alarms and more trust in the system.
If you are interested in learning more about how to identify false positives, it is important to know how AI detectors work.
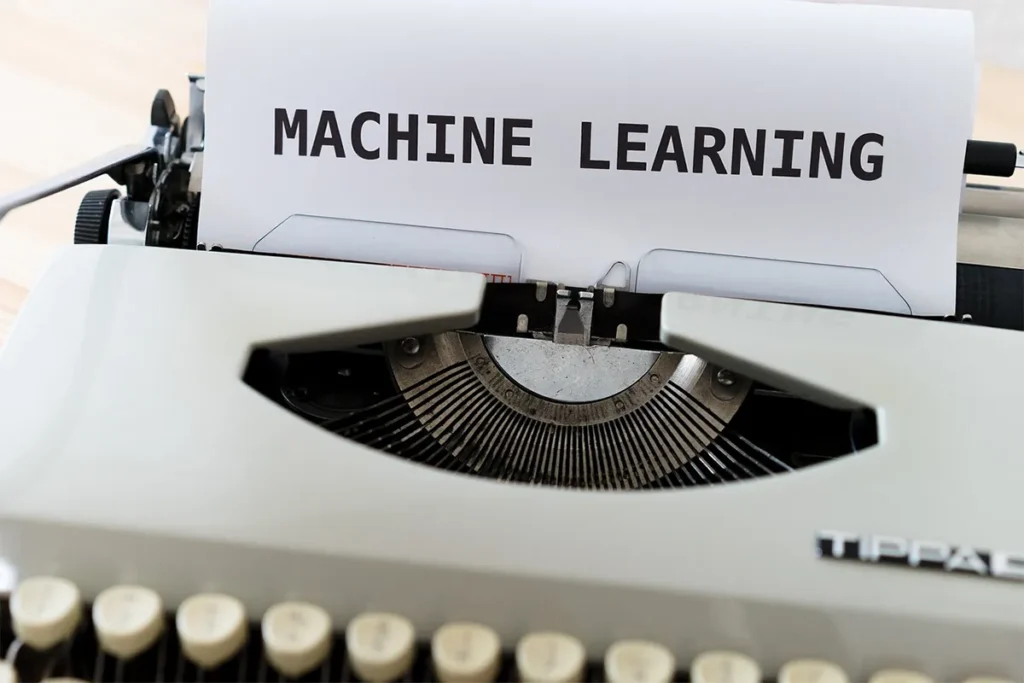
Strategies to Avoid False Positive
To prevent false positives from happening, strong data preparation is necessary. Improving training datasets can affect the output of the model. When the datasets are rich and diverse, AI learns better. Implementing data quality controls checks the accuracy of the information used.
Users must also think about improving algorithms. Using more sophisticated models can enhance performance. Regular updates and retraining ensure that the system evolves with changing data trends. Monitoring and feedback loops play a big role, too. They provide feedback on performance. With this method, models can adapt based on real-world performance. This constant adjustment helps to reduce the possibility of false positives.
Tools and Techniques to Escape False Positive
Tools are available to help reduce false positives in AI detection and to eliminate the associated AI risks. AI-powered monitoring tools can analyze large amounts of data. They learn from past errors and improve over time. These tools help organizations find problematic content with better accuracy. They help to identify real threats and ignore safe information. Companies use these tools more and more for better results.
Predictive analytics platforms play an important role. They look at patterns in data. These platforms can find warning signs. They do this before false positives happen. By understanding the data, businesses can make better decisions. This insight can make AI detection systems more effective.
Future Trends in AI Detection Technology
Many future trends appear in AI detection technology. One trend is advanced machine learning algorithms. These algorithms analyze data in complex ways. They can see small differences. Simpler models may not see these differences. This ability can lower the number of false positives a lot.
Another trend is using human feedback in AI systems. Human insights help machines learn. This teamwork makes a more accurate detection system. AI helps human judgment. AI should not replace human judgment. Combining both can improve performance in many areas.
Conclusion
The last point is about continuous improvement in AI detection. Organizations should not accept average performance. They need to refine their systems all the time. Regular updates and evaluations lead to better accuracy. Each small improvement makes significant changes over time.
Stakeholders in AI development and implementation have a critical role. They should make sure their tools work well. They should also reduce risks. Investing in better technology and practices helps everyone. The idea of perfect AI systems may not be too far away if we commit to these actions.